Slot Gratis
Demo Slot: Link Slot Online Gratis No Limit, Pragmatic Play Demo Rupiah
Demo Slot: Link Slot Online Gratis No Limit, Pragmatic Play Demo Rupiah
Couldn't load pickup availability
Demo Slot: Link Slot Online Gratis No Limit, Pragmatic Play Demo Rupiah
Demo slot merupakan game percobaan gratis yang dihadirkan oleh pragmatic play demo. Slot demo dapat dimainkan dengan cara gratis yang dimana para slotmania tidak perlu melakukan deposit. Demo slot juga memiliki dua jenis mata uang sekaligus yaitu rupiah (idr) dan dollar (usd). Yang perlu dimainkan oleh slotmania tentu pragmatic play demo rupiah. Sesuai dengan mata uang tanah air tentu akan lebih gampang memainkan game slot demo. Untuk hal ini pastinya para slotmania harus memilih link slot demo resmi. Dengan memainkan pada link slot demo gratis para slotmania bisa mendapatkan credit no limit. Menariknya lagi, semua demo slot mirip dengan asli sehingga tidak akan terasa perbedaannya saat bermain slot uang asli. Tentu ini menjadi sebuah inovasi terbaik dari pragmatic play demo untuk para slotmania. Sebelumnya itu, para slotmania harus mendaftarakan akun demo slot terlebih dahulu. Mengenai akun demo slot bisa didaftarkan gratis melalui website resmi pragmatic play demo.
Share
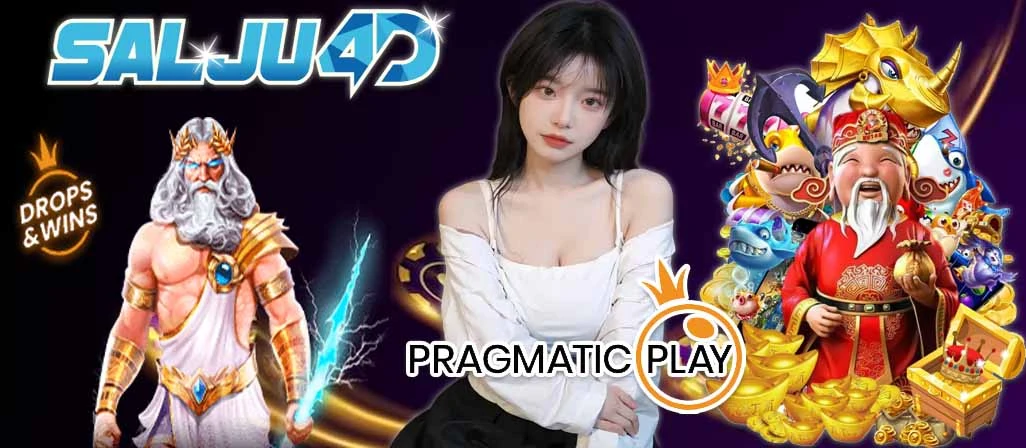